Enabling Broader AI and ML Analytics Adoption: Data Platforms, Tools, and Practices
Enabling the broader adoption of AI and ML analytics use cases is one of the top drivers behind the modernization of data infrastructure at companies right now. From gathering, transforming, and processing data to the development, testing, and monitoring of data pipelines and models, deriving greater business value from AI and ML initiatives requires the right mix of platforms, tools, and practices.
To be successful, companies need a modular data architecture that can quickly accommodate new use cases. They also need the ability to rapidly integrate data, well-defined data governance and security processes, and standardized processes for building data pipelines. The use of automation, MLOps and AIOps methodologies, and cloud services that offer on-demand scalability are all key pillars for success as well.
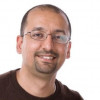
Chief Technology Officer
Lalit Ahuja is responsible for the direction, implementation and delivery of GridGain’s technology strategy. He works closely with GridGain customers and partners worldwide and leads the Engineering, Product Management and Delivery teams in ensuring GridGain products and services meet or exceed all customer expectations.
In his career spanning over 25 years, Lalit has led various IT functions including enterprise architecture, product management, large scale program management, IT operations and served as a digital strategy advisor to executives at Fortune 500 enterprises.
Prior to GridGain, Lalit led the global Customer Success team at Akana, a leader in API Management and Application Services Governance space, where he was responsible for increased customer retention and more than 300% growth in Akana’s consulting services business.
Lalit holds a Master’s degree in Engineering and an MBA from UCLA.